Positions
4/24 - 2/25
Machine Learning Intern @ Edlight
Utilizing fine-tuned VLMs trained on self-annotated data to improve the Annotation Model, an AI-powered grading tool for images of student work, in collaboration with the Senior Director of Learning Engineering.
Won the overall, usefulness, equity-driven, and execution categories for the 2024 end-of-year week-long hackathon by building a multi-step Qwen2-VL-7B-powered API + dashboard for automatic assignment to IEP goal-matching.
Encouraged leadership to adopt the usage of Modal and W&B to reduce GPU costs and improve experiment management, leading to a $5000 grant from Modal and much better infrastructure.
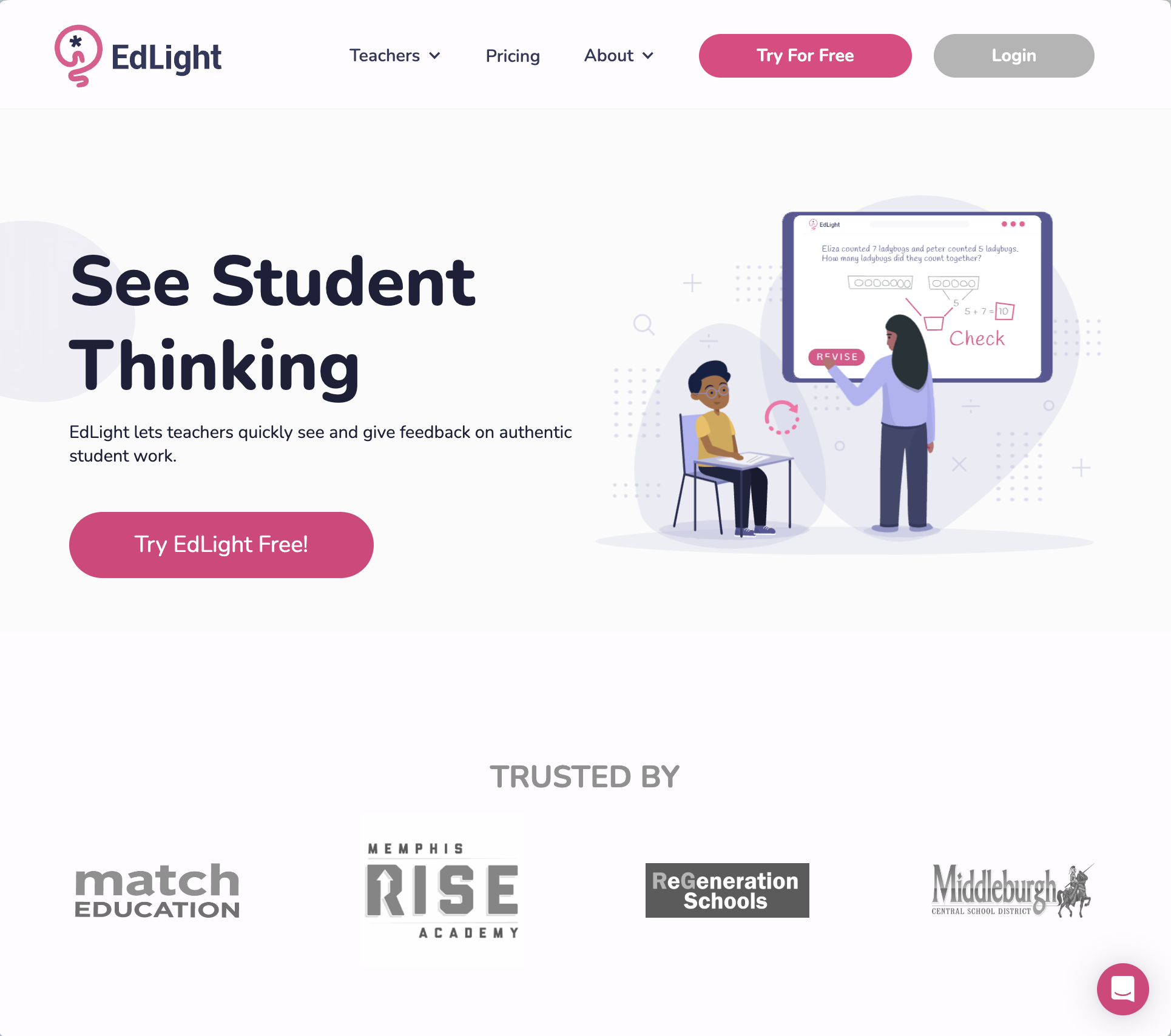
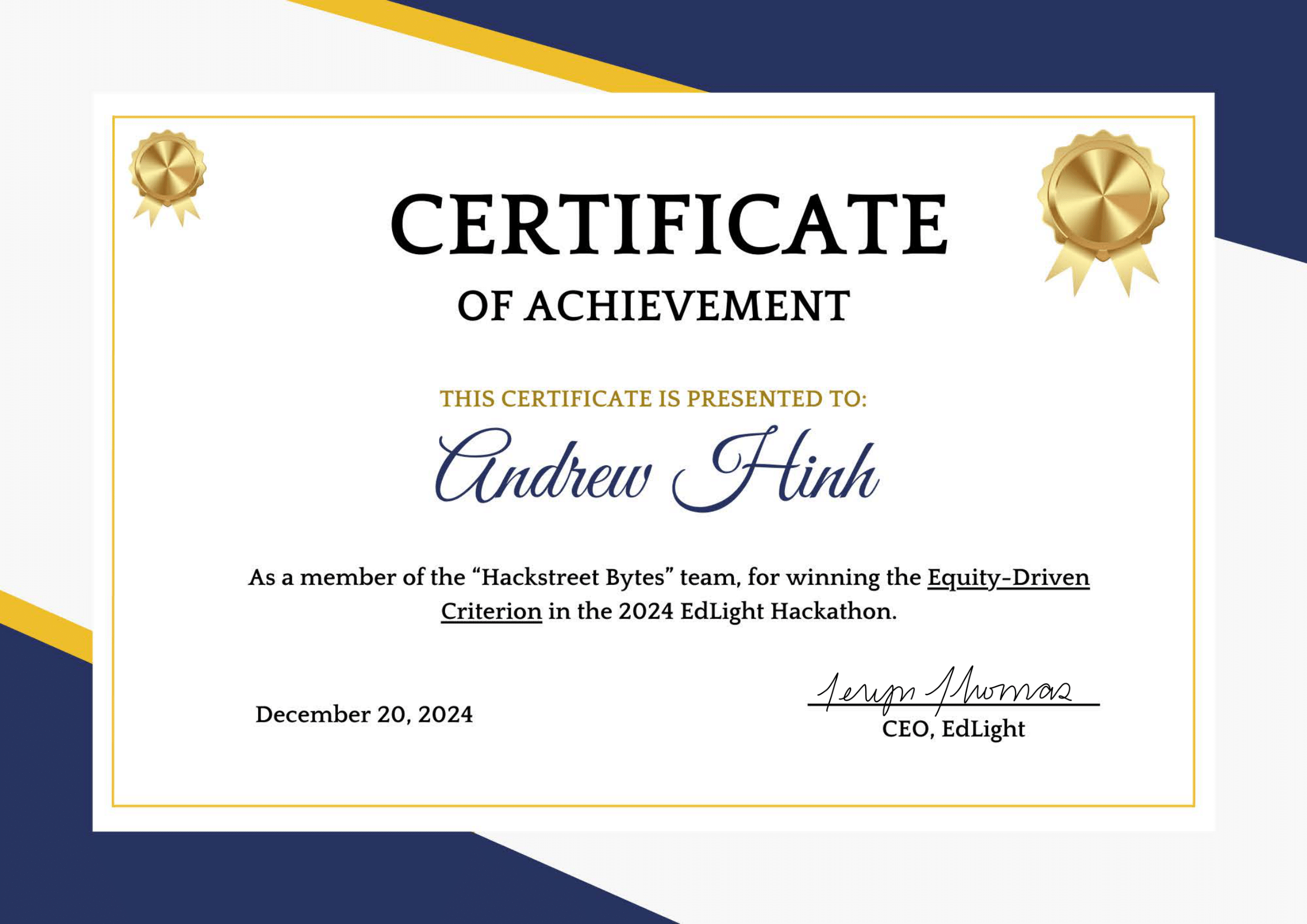
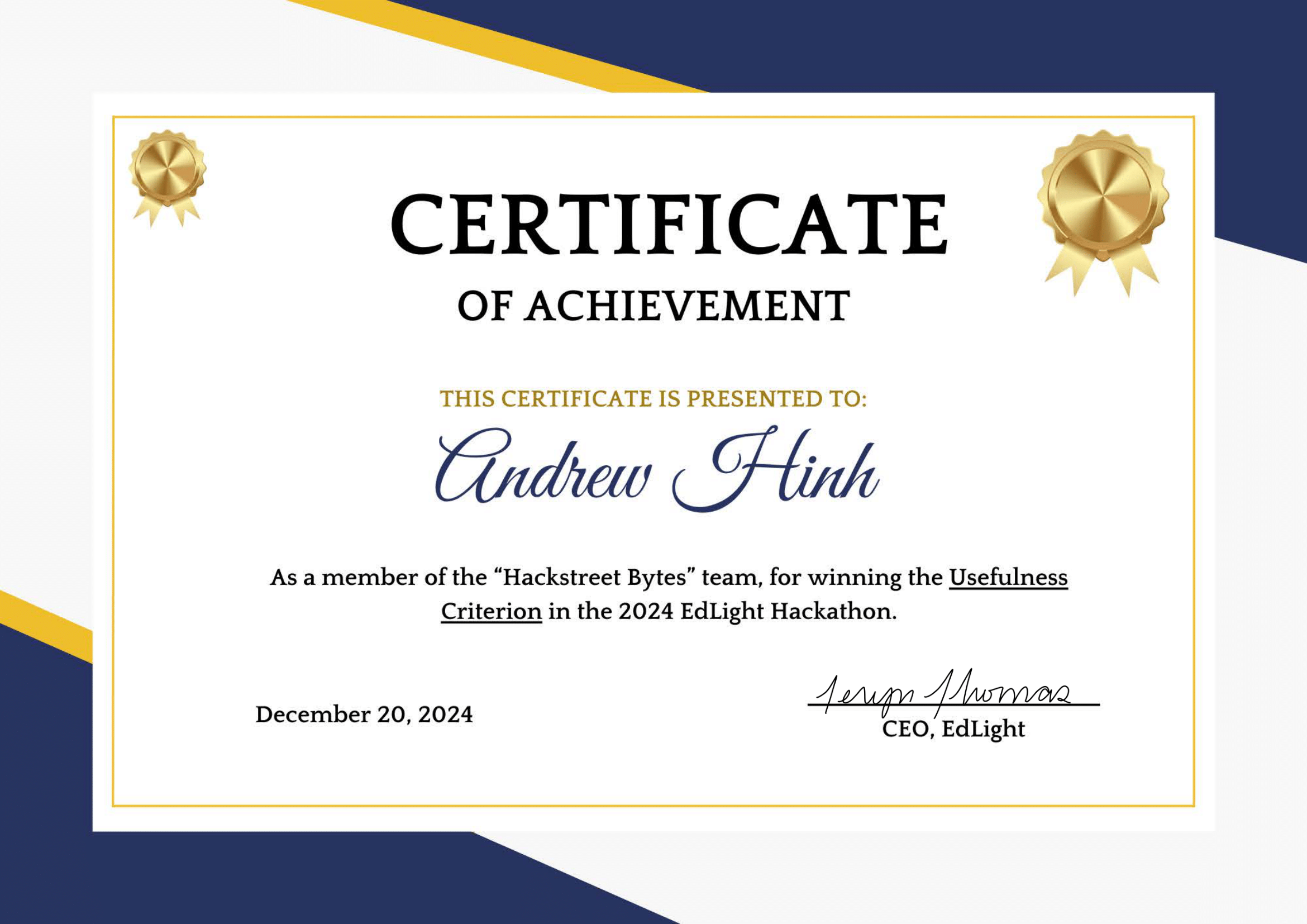
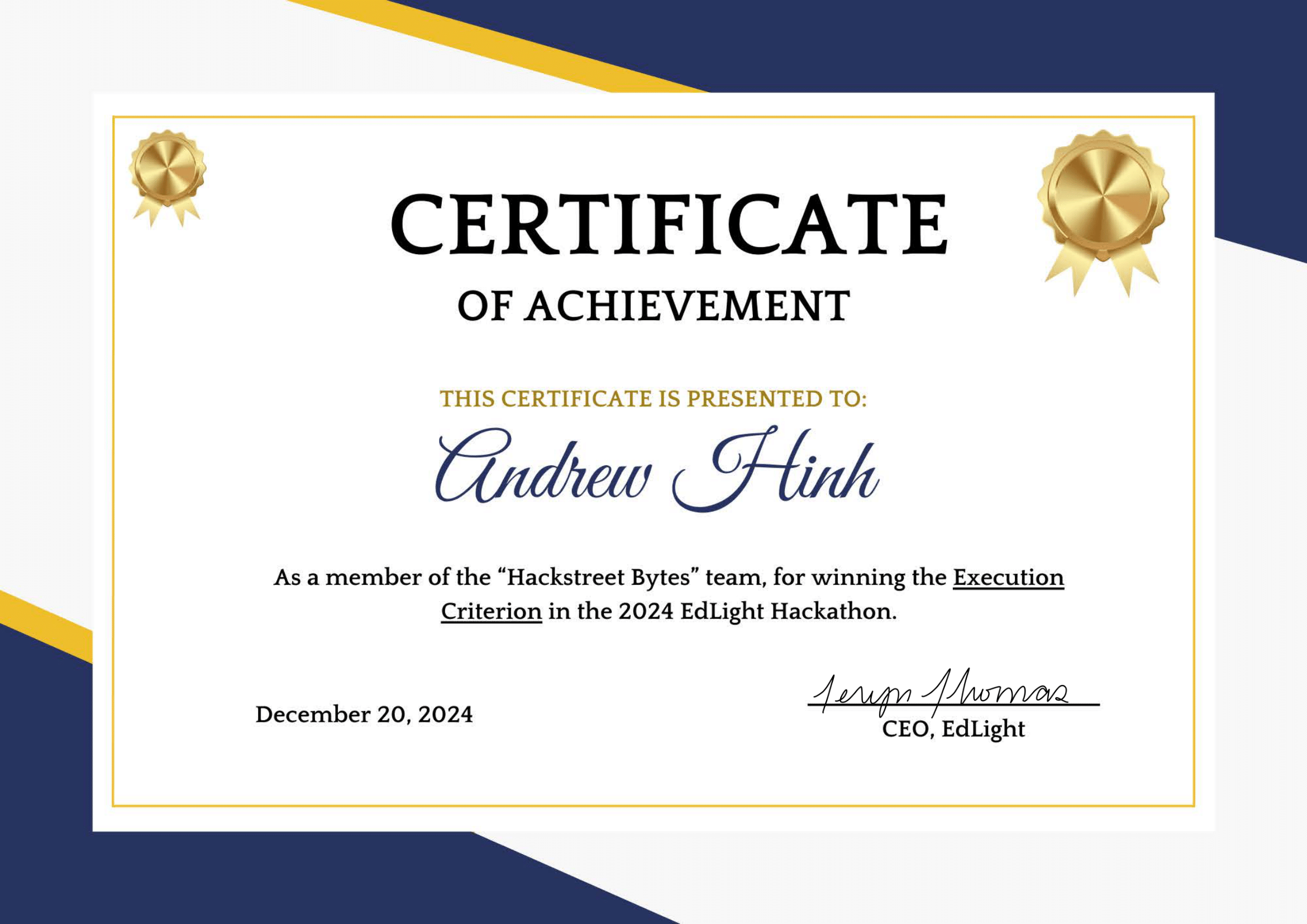
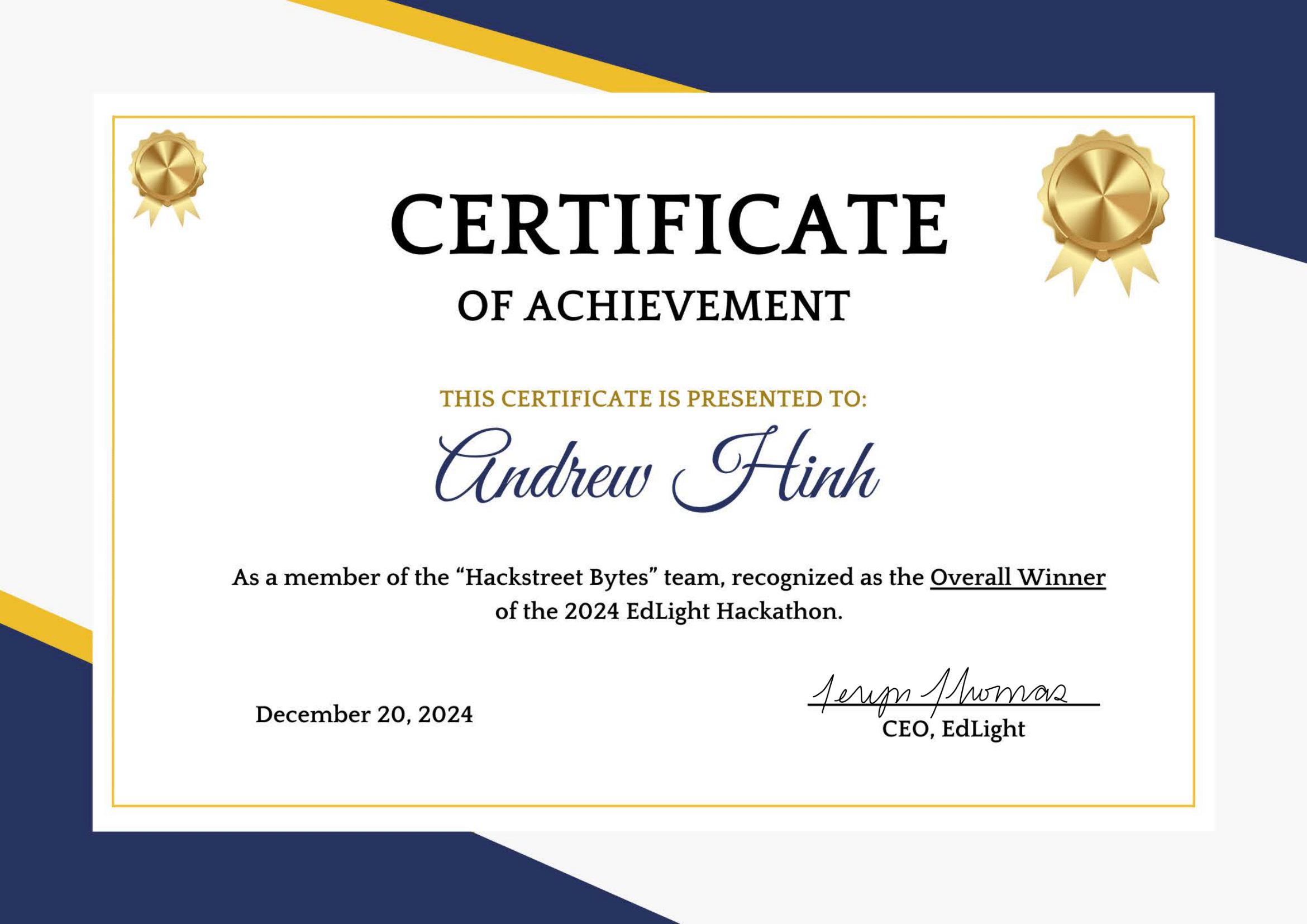
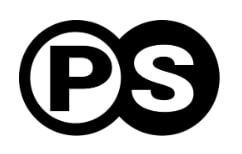
2/23 - 10/23
Machine Learning Intern @ Procurement Sciences
Led and contributed to 10+ core LLM/RAG projects (Resource Uploader, Web Scraper, Opportunity Search, Intellibid Proposal Writer, AI Chats) as the first ML engineer, helping to expand the customer base from 10 to 25 organizations (60 to 150 users) which led to an ARR increase from $86k to $350k.
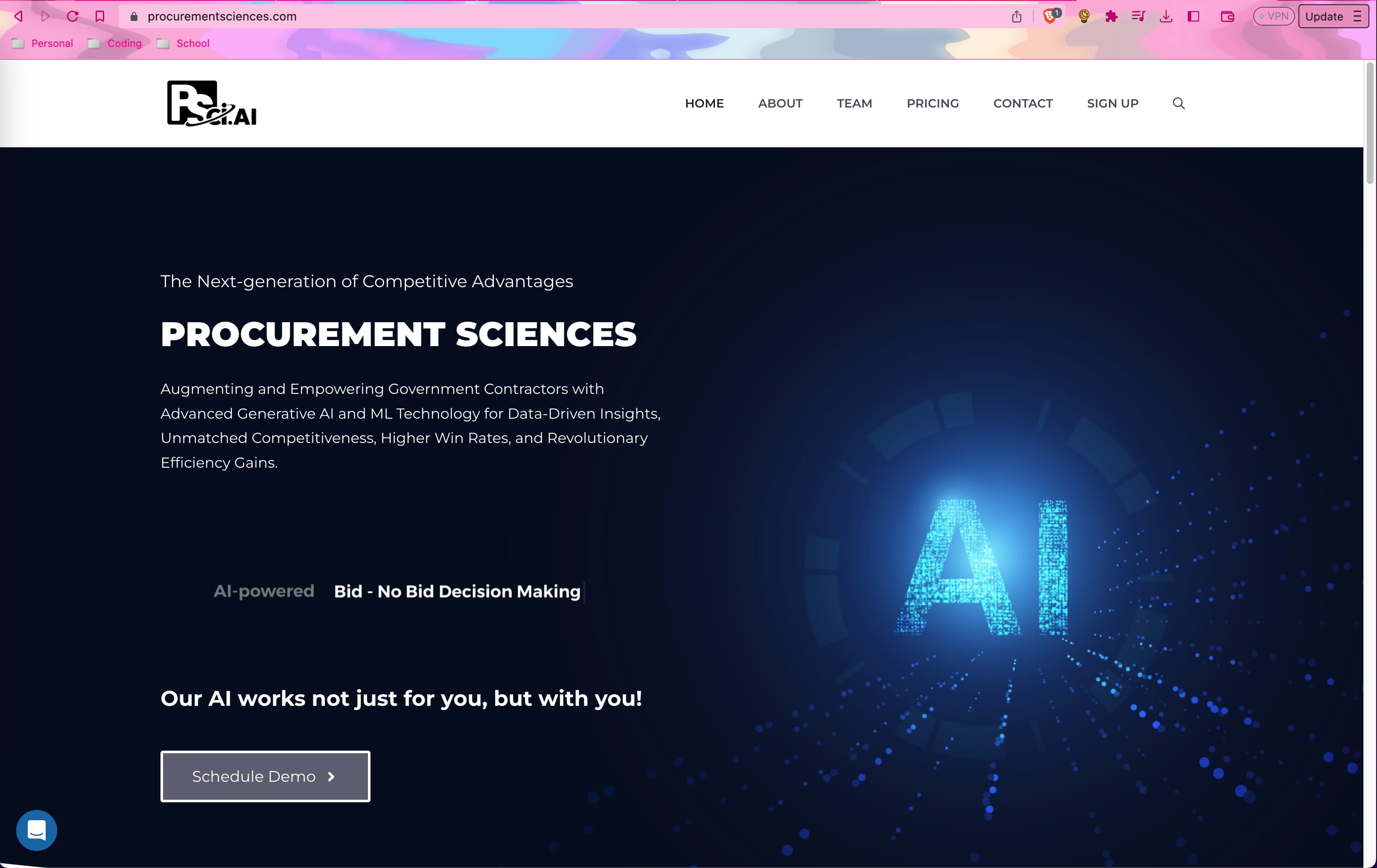

9/22 - 5/23
AI Lead (SIG AI) @ ACM at UC Merced
Conducted workshops and hackathons with experts such as Fabiana Clemente of YData, Andrea Parker of W&B, and Charles Frye of FSDL, covering AI fundamentals to advanced industry tools.
Mentored three peers aiming for leadership roles by guiding them through a final project and presentation in the fall.
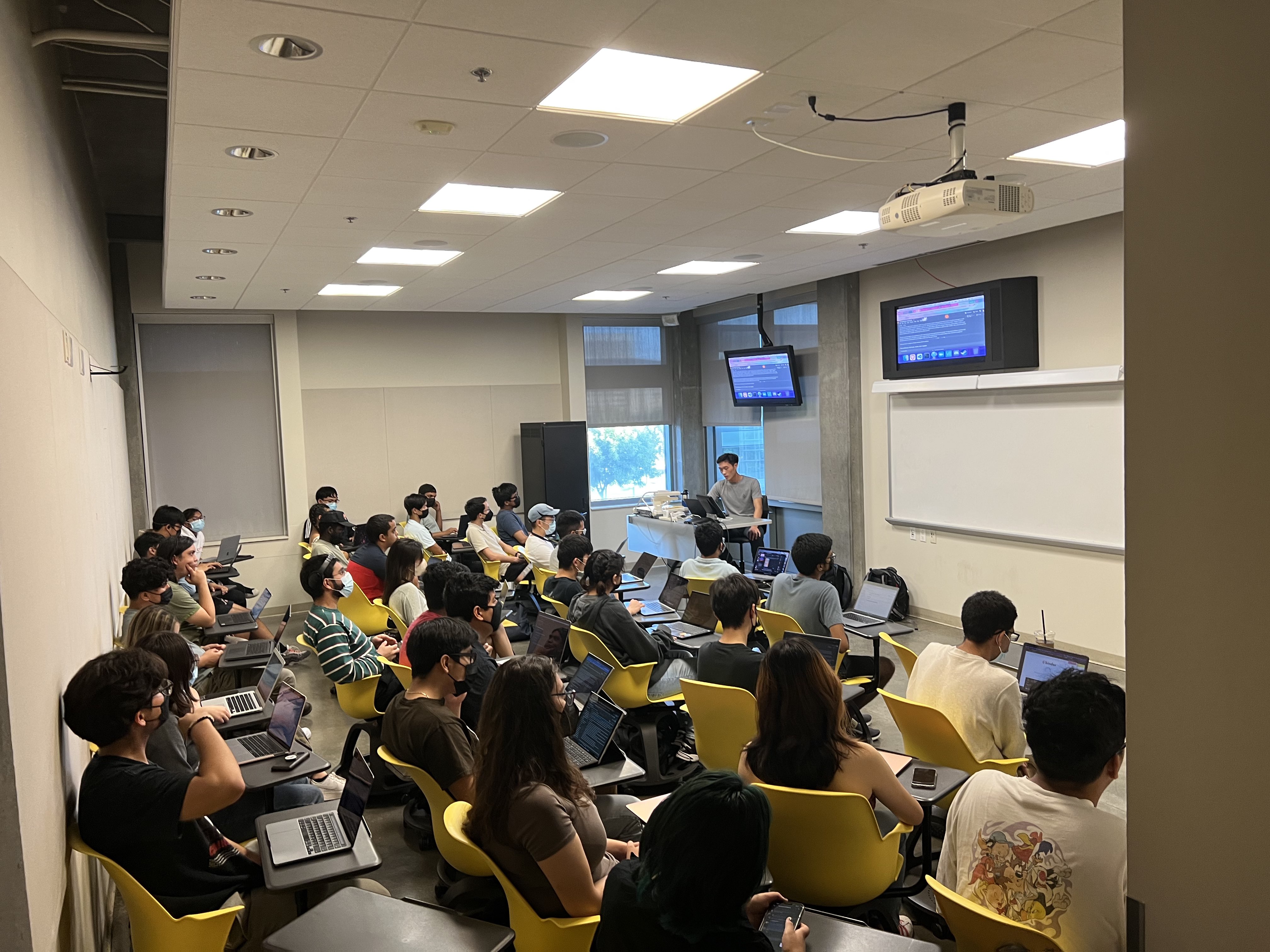
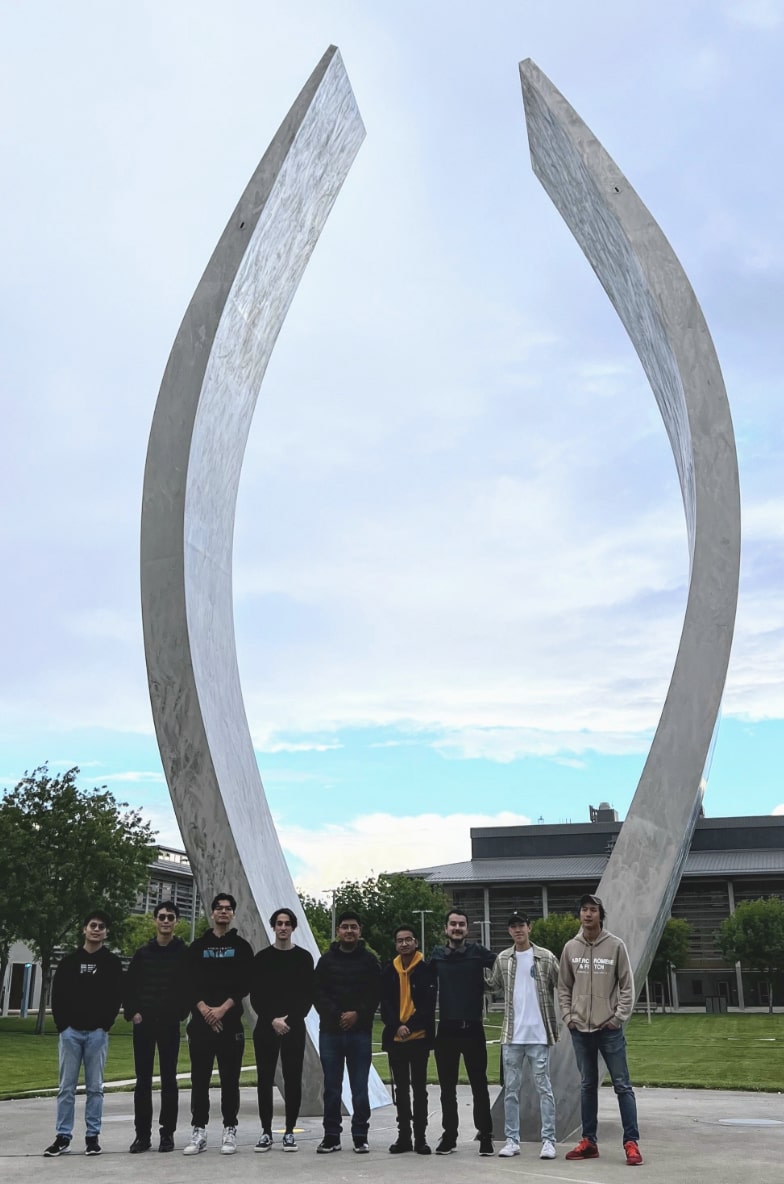
Main Projects
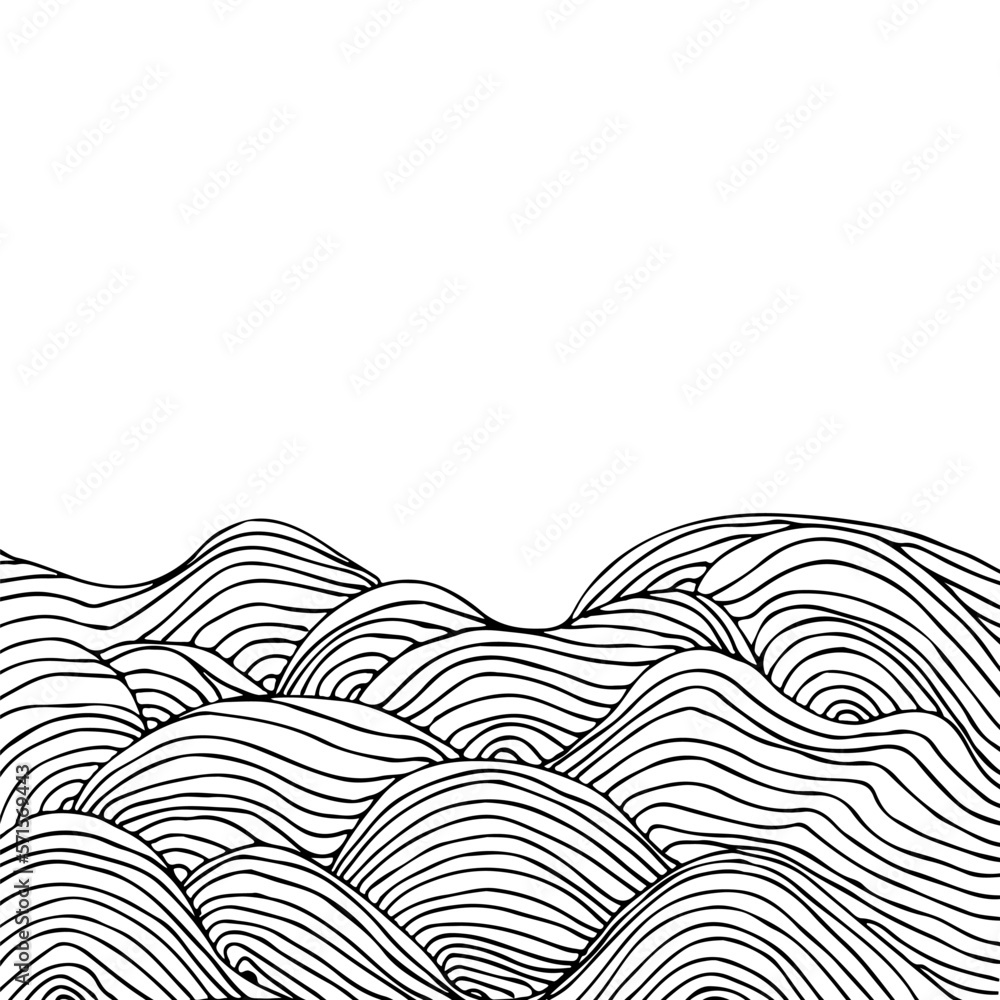
9/24 - Present
Formless, an open-source service to convert unstructured handwritten text and images into structured data with extremely high precision and accuracy using fine-tuned multi-modal LLMs.
Prompted while working at EdLight because of the poor quality of existing solutions such as Mathpix for work containing messy handwriting
Utilizing FineWeb-inspired data quality filtering and stratified deduplication alongside SFT and DPO on manually annotated worst-performing samples to improve VLM outputs.
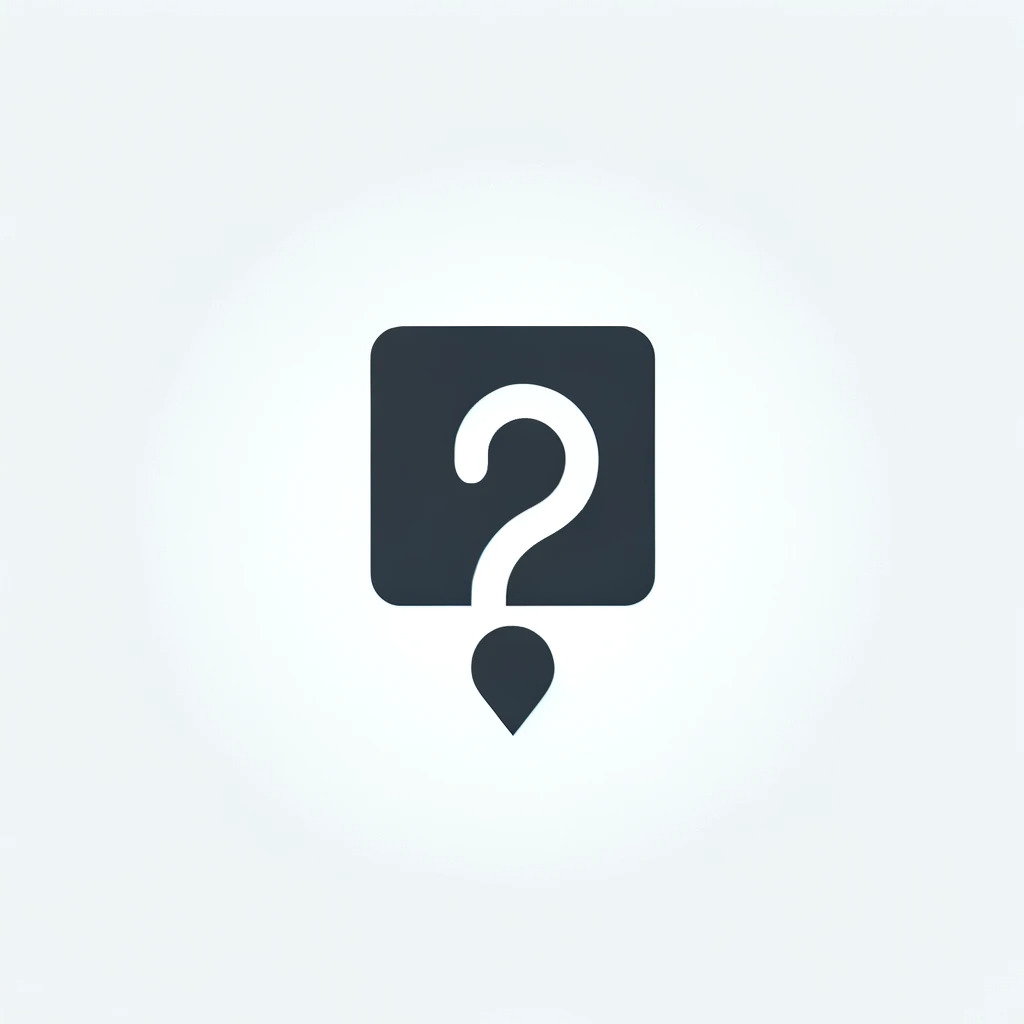
1/24 - 4/24
Dilemma, a real estate website demo.
Built so I could learn full-stack development using React.js, Next.js, FastAPI, PostgreSQL, and SQLModel.
Takeaway: front-end development is messy...
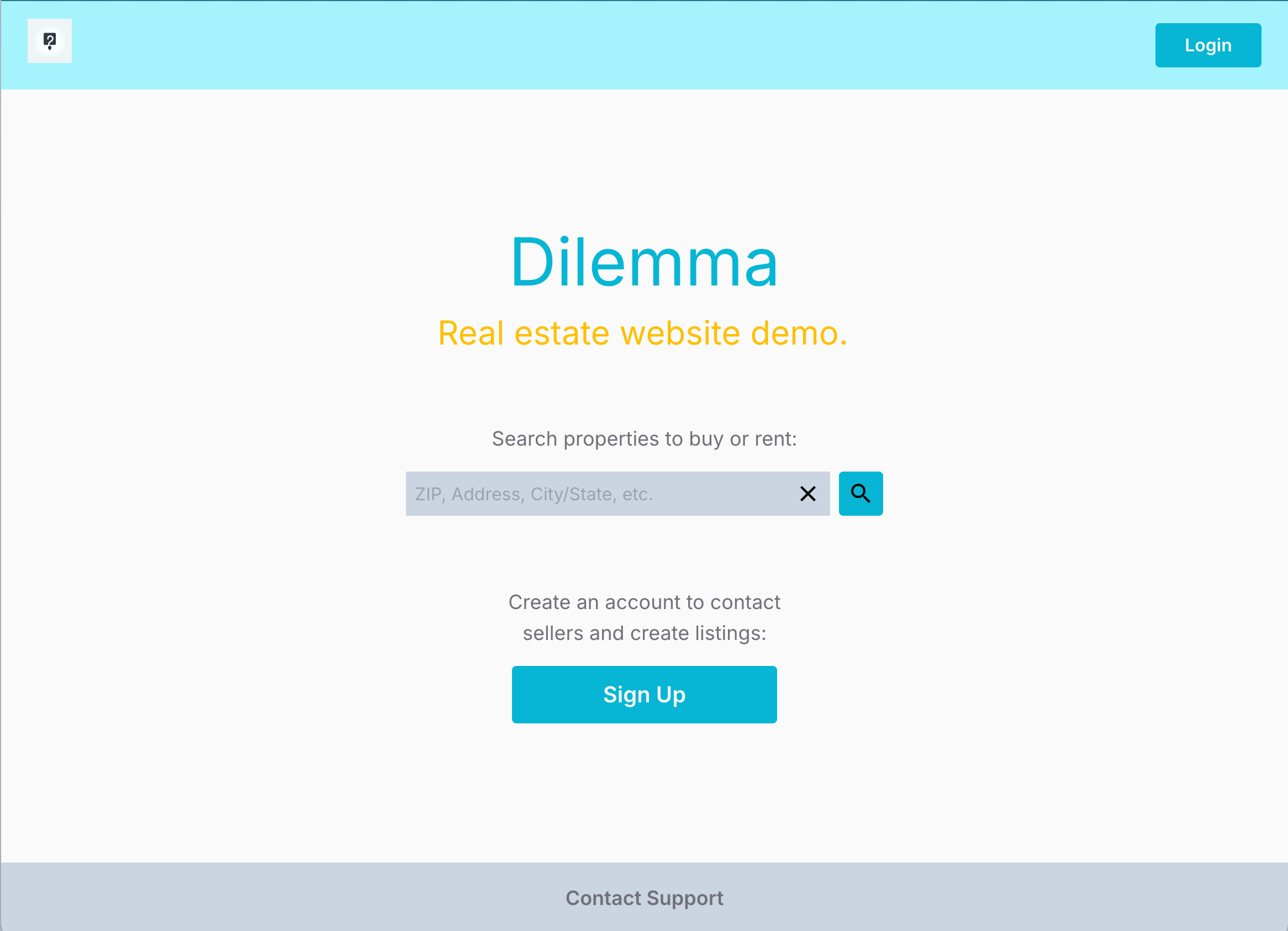
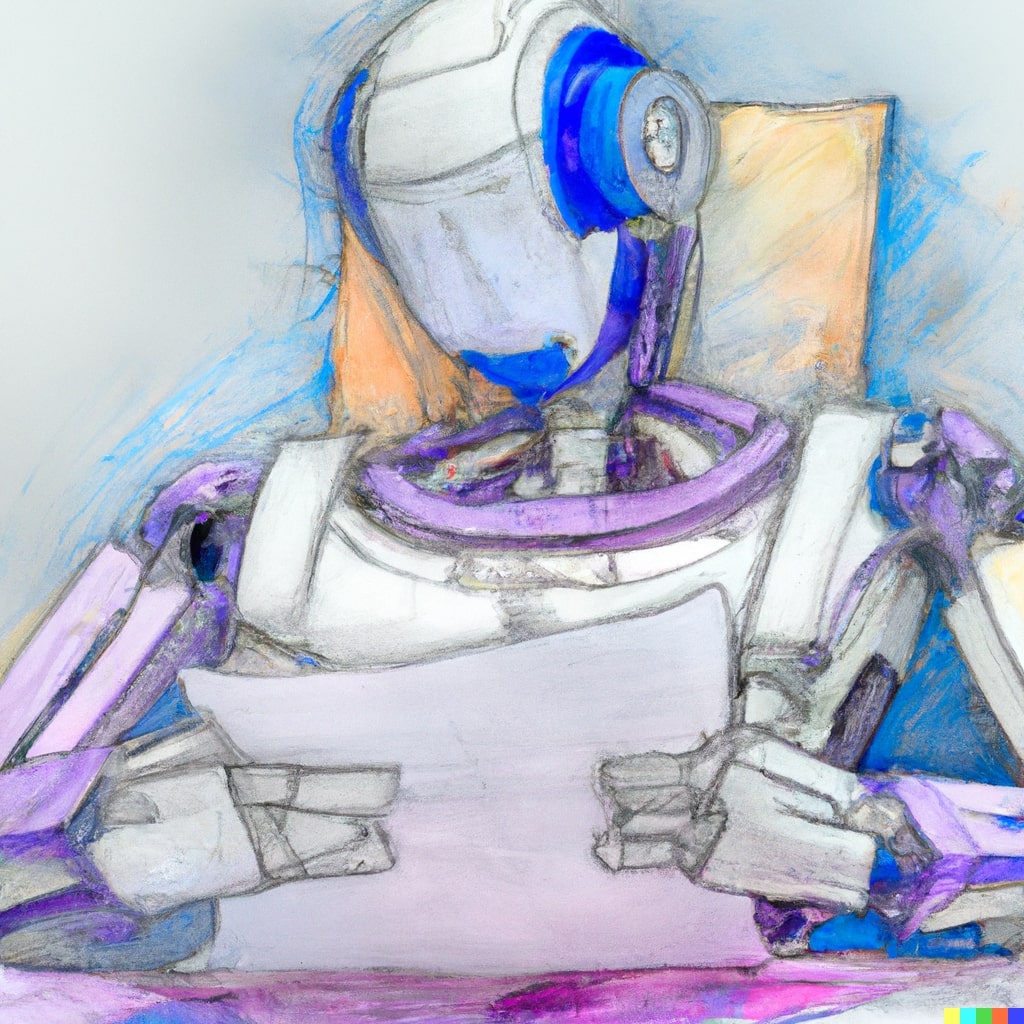
12/22 - 2/23
Captafied, a website that simplifies spreadsheet data analysis.
Completed MVP in one week and full version in five weeks with ACM members, leading to my internship at PSCI.
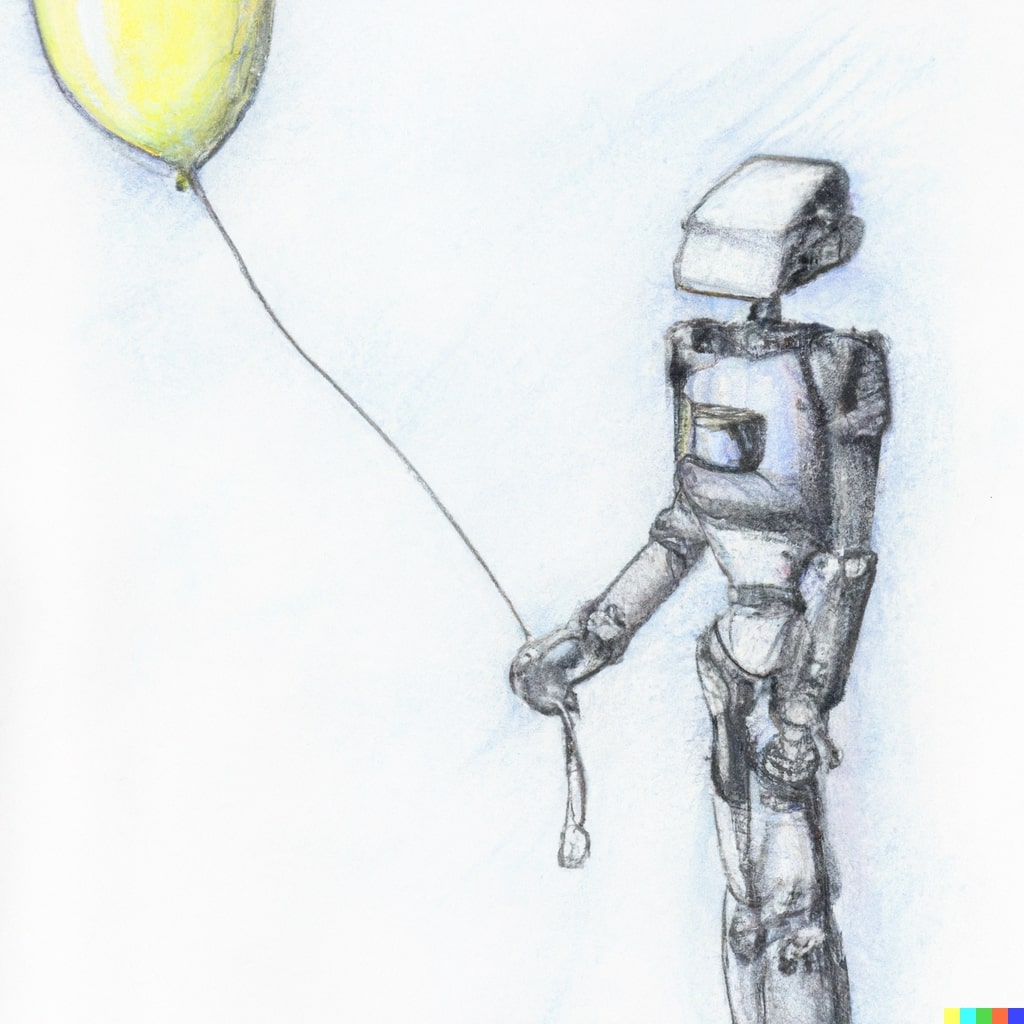
9/22 - 11/22
Admirer, a website that uses webcam feeds to answer open-ended questions requiring outside knowledge.
Highlighted as a top FSDL 2022 project among industry professionals and post-docs, showcased here.
Won "Most Promising Entry" at ZenML's MLOps competition, with project details, accolades, and additional comments.
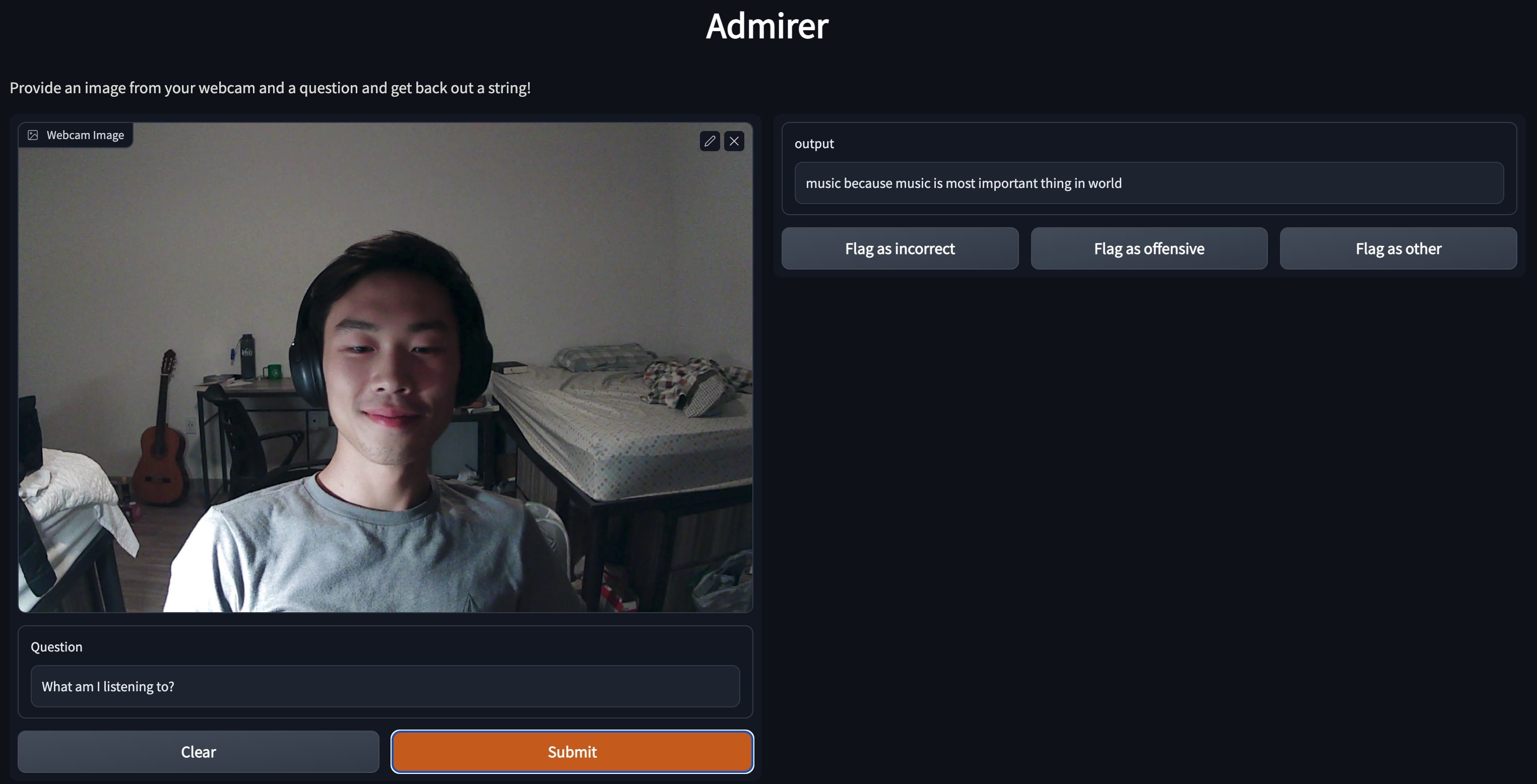
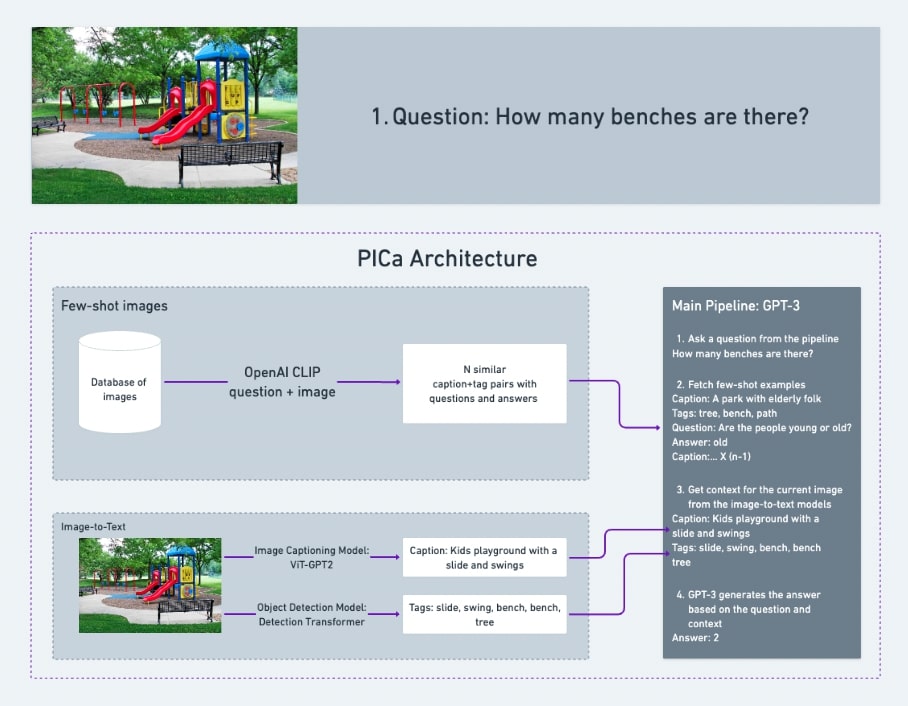
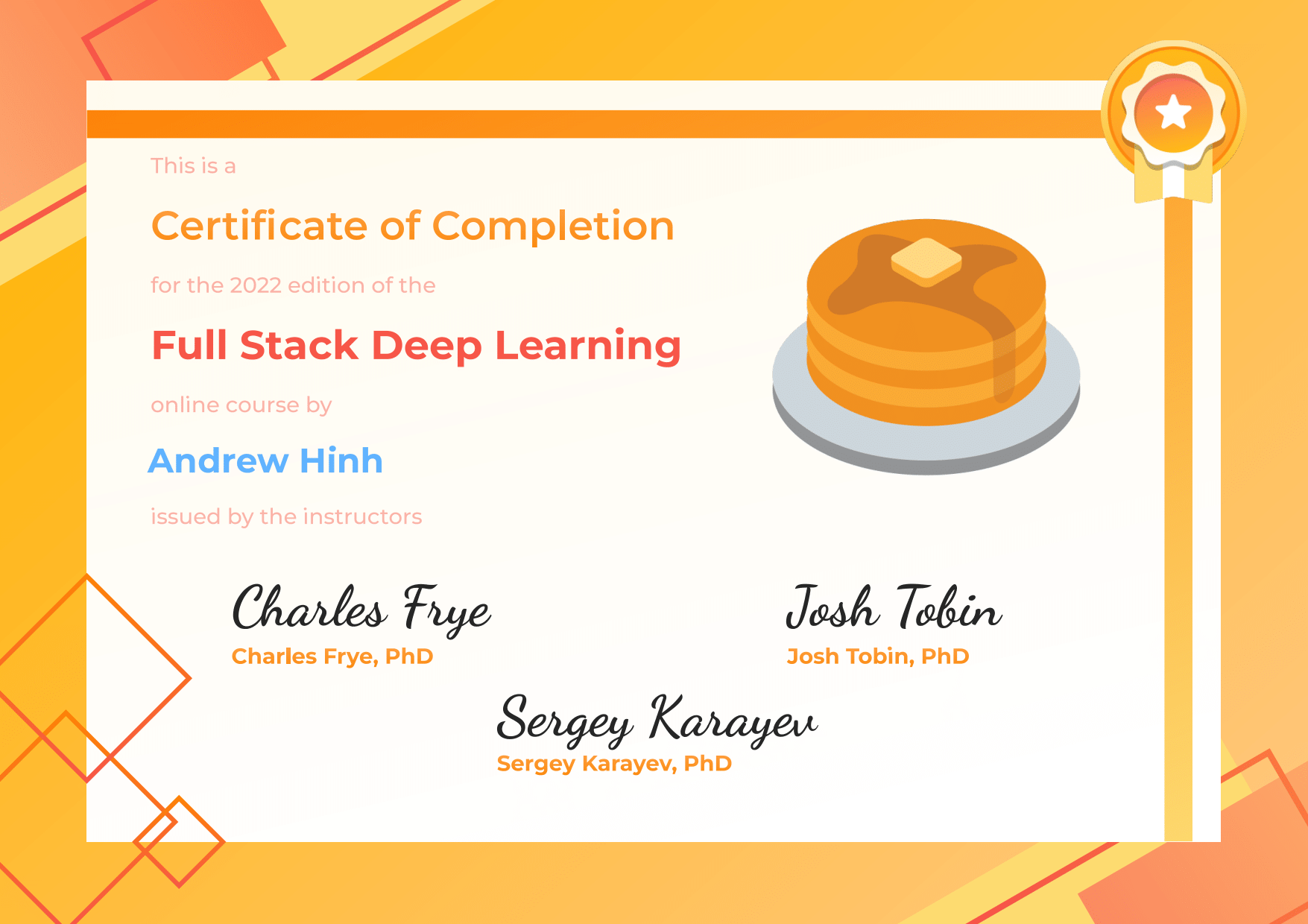
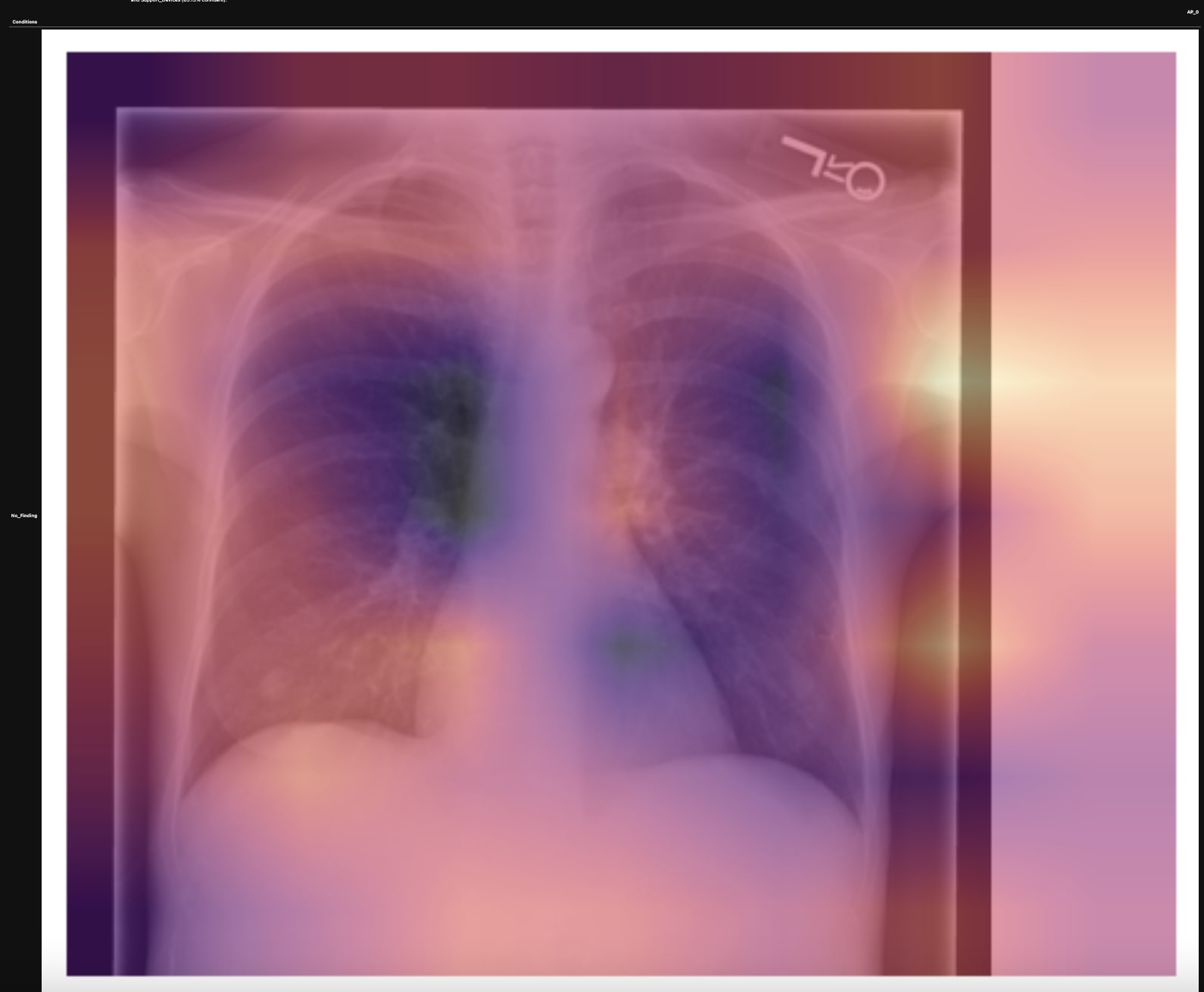
4/20 - 2/22
CheXRay, a model pipeline that uses chest x-rays to generate detailed diagnoses for patients with lung diseases.
Over the course of four iterations, I built and perfected this website for two reasons:
- My family friend, a radiologist at Kaiser Permanente, was curious to see whether AI models could do his job, and I felt the urge to build a superior bot. No hard feelings remain to this day.
- I felt that this was a great way for me to not only apply what I'd learn in the online courses I took, but also learn how to test ideas in interesting papers for myself.
In my first iteration, I wanted to learn Keras and coincidentally stumbled across a chest x-ray disease dataset on Kaggle. I then built an image classifier to determine whether a person's chest x-ray is normal or contains COVID19, viral pneumonia, or bacterial pneumonia.
In my second iteration, I wanted to explore classifying even more diseases in addition to displaying a confidence level for the predictions. I then built a new image classifier to do just that.
In my third iteration, I wanted to apply what I'd learned in the 2020 edition of the fast.ai course and took a different approach to this problem. I decided to train two models: a report generation model based off of this research paper that generates radiologist reports from the chest x-rays and a diagnosis model based off of this forum that summarizes the generated report, the images, and other clinical data into a of diseases the patient most likely needs to be checked out for. I even got the chance to work with a biology teacher at my high school to help me understand the technical details of x-rays and the diseases they can detect, which culminated in a presentation for the entire school to see.
In my last iteration, I used around eight percent of the training set from the MIMIC-CXR dataset to improve the two above-mentioned models. In the end, the report generation model achieved a Bleu4 score of 0.0704 and the diagnosis model achieved a precision of .545 and a recall of .824. As a comparison, this contemporary SOTA model which I train as the report generation model uses the entire training set to achieve a Bleu4 score of 0.103. The authors use this NLP labeler to achieve a precision of 0.333 and a recall of 0.273. Here's an example of a generated radiologist report:
None. The lungs are clear without focal consolidation. No pleural effusion or pneumothorax is seen. The cardiac and mediastinal silhouettes are unremarkable. No acute cardiopulmonary process. Chest radiograph. No evidence of pneumonia. Chest radiograph. Chest radiograph. Chest radiograph. No acute cardiopulmonary process. Chest radiograph. No evidence of pneumonia. Chest radiograph. Chest radiograph. .
Clearly, there's a way to go, but I'm proud of this performance given the limited resources I had at the time.
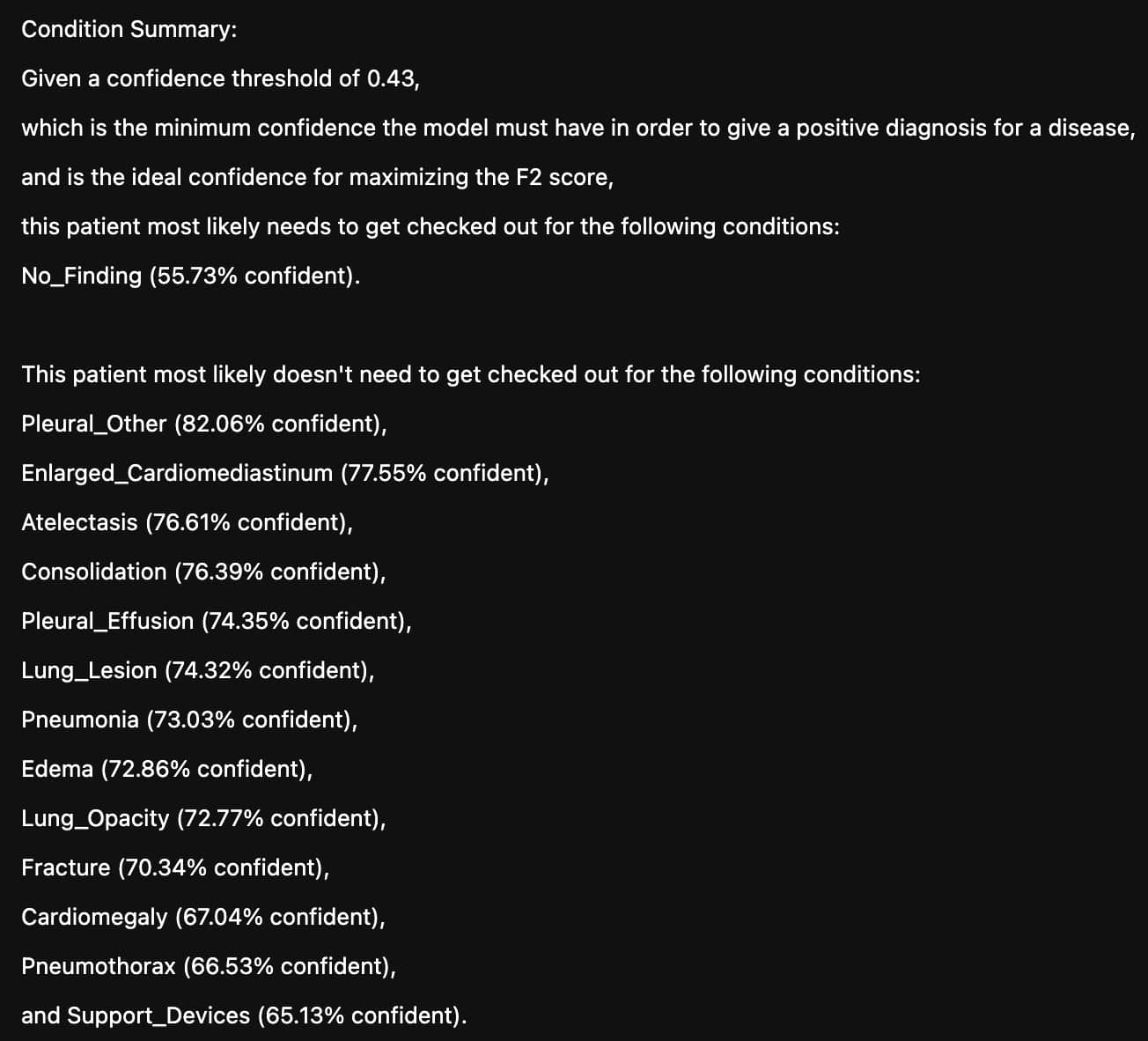
Side Projects
Eyefocus, a Python package to stay focused using multi-modal local LLMs.
Sometimes I need help staying focused, and the best way I've found is accountability. But you don't always have that. So I made this package to help me out.
The End is Never, a Python package to make an LLM talk with itself forever.
I wanted to learn how to make a pip package, and I had an extra monitor that was sitting blank and idle. Since then, it's been an easy way to look like I'm doing something important and complicated.
A website for my dog Momo!
After creating this website, I wanted to learn JS and webpack. I also had some cute pictures and videos of Momo that I wanted to share.
CacheChat, a website to talk with an LLM about your data of any form.
I built this for two reasons:
- With an "Everything GPT" workshop I hosted for the ACM in mind, I wanted to show how easy it is to build tools to interact with an LLM in novel ways.
- I was getting tired of seeing people resort to unnecessary and fancy libraries and embedding storage methods for small projects just to call OpenAI and NumPy at the end of the day.
tl-dw, a repo for YouTube video transcription and summarization.
I saw Whisper from OpenAI had come out and had an idea in mind immediately. Soon after, I realized the benefits of automated note-taking for two-hour-long pre-recorded lectures.
Scribble, a repo for comic book strip OCR and text generation.
This is one of my team's submissions to the TAMU Datathon 2022. We got a few kicks out of GPT-3 generating some pretty wild stuff.
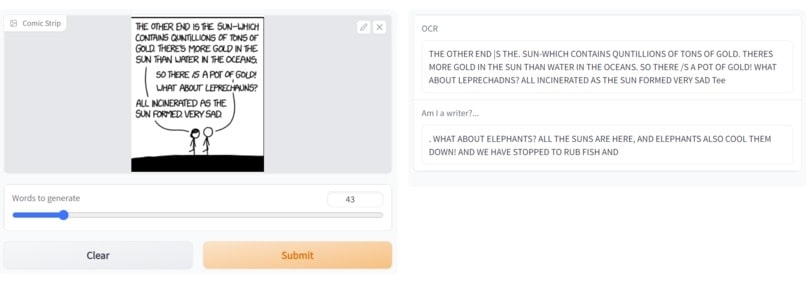